What Is Predictive Analytics?
Predictive analytics use algorithms and machine learning to anticipate future outcomes based on data. A form of predictive modeling, predictive analytics is the branch of big data analytics that is specifically concerned with revealing insights on what will happen in the future based on what has already occurred in the past. More accurately, it is concerned with what is likely to happen, since predictive analytics is not akin to having a crystal ball.
Predictive analytics has widespread applications across industries, from retail to healthcare and other sectors. In healthcare, for example, predictive analytics is increasingly used to anticipate patient outcomes and health risks. Regardless of industry, predictive analytics is one of the wide-ranging use cases for data science and machine learning in businesses and other organizations.
How Do Organizations Use Predictive Analytics?
First off, how do organizations use predictive analytics? Organizations make use of predictive analytics in one of two ways: direct and indirect.
The direct use of predictive analytics involves data analysts, business analysts and data scientists who source and curate large data sets and implement predictive models against that data. These roles may consist of full-time employees, as well as outside consultants and agencies. The team may be supplemented by database administrators and software engineers.
The predictive analytics team may use open source tools, commercial tools (e.g., SAS) and tools that they develop in-house. The predictive models use a combination of mathematical and artificial intelligence methods including linear and nonlinear regression, neural networks and decision trees.
The predictive models help the organization forecast future events and behavior based on historical data. Forecasted events include customer purchase activity and customer churn. Scientists can use predictive models to forecast weather trends, climate change patterns and more.
The indirect use of predictive analytics is far simpler: organizations deploy software tools and platforms for which predictive analytics is a core or secondary built-in capability. In this scenario, organizations can benefit from predictive analytics without needing to understand its inner workings.
For example, a marketing automation platform might provide predictive lead scoring as a key capability. The marketer using the platform knows that lead conversion rates improved, without knowing that the result is due to predictive analytics running “under the hood.”
Predictive Analytics in Retail
The retail industry is highly competitive, where brand and price are important, and customers are unpredictable. Given retail purchasing volumes, small gains in purchase affinity can result in millions of dollars (or more) in sales gains. This is where predictive analytics in retail comes in.
The effectiveness of predictive analytics hinges on the volume and quality of the underlying data. In retail environments, an abundance of consumer data is generated. On an e-commerce website, customers generate data from clicks, purchases, shopping cart abandonment and more.
In a physical store, data is generated from royalty programs (i.e., detailed purchase history) and from RFID data (when enabled) that captures shopper movement through the store. Shopper movement is also tracked by some stores using video surveillance data.
Predictive analytics uses these rich data sets to predict future customer behavior. For example, models might predict that a particular brand of cereal will become popular with shoppers. A store might respond by offering a limited time price cut to drive even more sales. Or, they might move those cereal boxes to the endcap—the shelf placed at the end of an aisle. By using an insight generated by predictive analytics, retailers can maximize the associated increase in sales.
Predictive Customer Analytics
If you’re a regular shopper at Amazon, you’ve seen recommended products that you didn’t know you needed. Upon reflection, you decide you do need those products and buy them.
That’s predictive analytics for consumer behavior at work, where data models forecasted your current and future needs before you even realized them. In addition to offering products to purchase, predictive analytics can identify customers at risk for churn. In response, these customers could be offered discounted renewal prices or be contacted by a customer support or customer retention specialist.
Predictive analytics can also forecast future product sales and customer demand, enabling organizations to have adequate inventory available and sufficient support personnel staffed. For example, a nationwide pizza chain can use predictive customer analytics to forecast how many pizzas will be sold during the Super Bowl and how many workers each location needs to staff.
Predictive Analytics in Marketing
To implement predictive analytics in marketing, the first step is to identify and gather the right marketing data. The data sets might include customer purchase data (e.g. product SKU, quantity, date purchased, price, sales channel, etc.), customer demographic and psychographic information and product return data.
The next step is data cleansing and data preparation. Data cleansing is a quality assurance step to ensure data is free of errors and that all mandatory fields are present. Missing values in key fields can compromise the quality of the predictive model. Data can also be enriched using data from partners and resellers and from third-party data providers.
The third step is to build the predictive models using data science methodologies like classification and regression. Classification is a process that assigns categories to a collection of data to allow for more accurate analysis. Regression models the relationship between a dependent variable and one or more independent variables.
The final step for predictive analytics in marketing is to expose or share the results. For example, a marketing automation platform that uses predictive analytics to create more meaningful lead scores would refresh the leads with updated scores. Learn more in our Marketer’s Guide to Predictive Analytics.
Predictive Sales Analytics
Predictive sales analytics can optimize the effectiveness and productivity of sales development representatives (SDRs) and account executives. Sales development representatives often deal with a list of 100+ leads. Predictive sales analytics can process these 100+ leads and prioritize them based on “best fit” as potential customers.
This lets SDRs focus their time on calling leads who are most likely to engage in a conversation on the phone or respond to an outreach email. In addition, using data from past interactions, predictive sales analytics can suggest the messaging (i.e., wording) that’s most likely to catch a prospect’s attention, as well as recommended content assets that are likely to advance the sale.
Predictive sales analytics can also optimize sales rep territory assignment. Rather than assigning sales territories based on geography, predictive analytics can assign territories based on where the best accounts are concentrated. This allows sales managers to distribute the best-fit accounts equally across the team, independent of where those accounts are located geographically.
Related Solutions and Resources
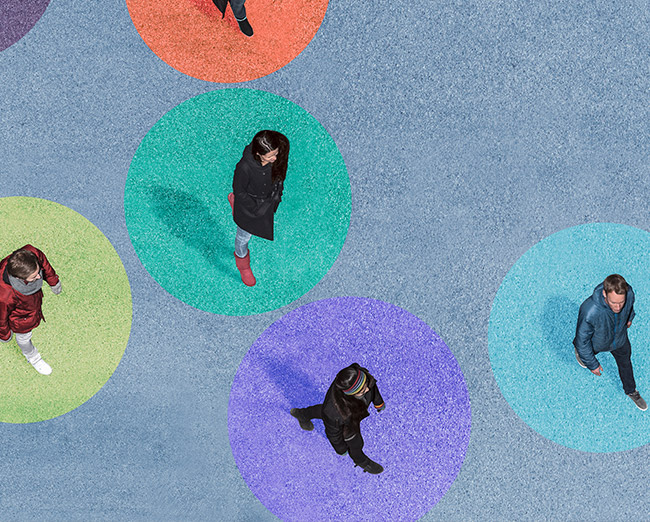
How Customer Data Helps Reinvent Retail for Social Distancing
Out of the Covid-19 crisis, a new playbook is emerging for socially distanced shopping, one that may help…
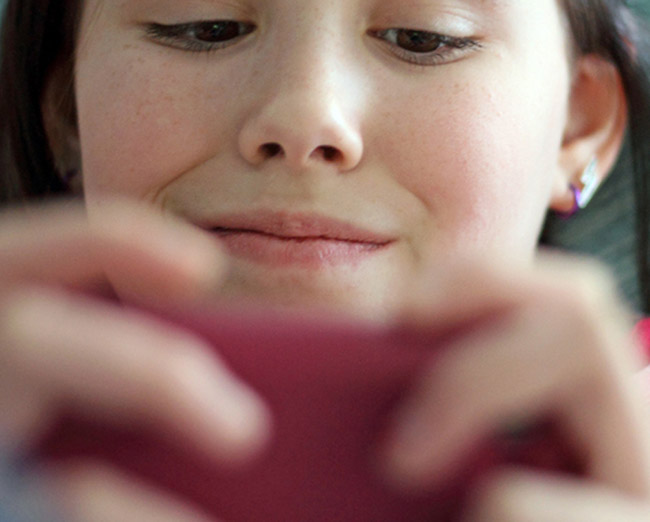
What Makes Gamers Stay? MobilityWare Uses CDP and Predictive Scoring for Answers
If you’ve ever played games on your phone, chances are you’ve played a MobilityWare game—either its…
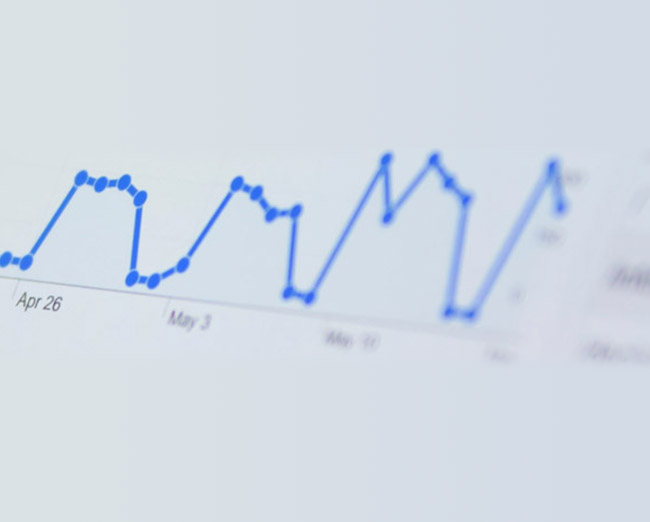
The Omniscience Option: Next-best-action Recommendations that Work
Next-best-action prediction is as close to omniscience as marketers are likely to get in this lifetime. Its aim…